Worsening macro-economic conditions around the world, coupled with increasing geopolitical tensions, as well as long-standing disputes in areas such as the South China sea, has a complex array of downwind effects on the maritime landscape. GSTS has a proven track record of delivering state of the art maritime Artificial Intelligence (AI) solutions designed to save lives, energy, and the environment. GSTS’ proprietary platform, OCIANA™ has been recognised for detecting maritime threats in coastal regions on a global scale. This whitepaper introduces the increasing complexity of maritime risk assessment and illustrates how combining novel AI methods with subject matter expertise provides the capability to detect and characterize subtle changes in maritime risk globally.
Across the board, the maritime sector has demonstrated concerns from a security perspective over the last few years, with an ongoing trend in reported piracy events, total cargo losses, and illegal fishing. There are several factors that have contributed to mitigating the negative effects including international cooperation, increased awareness, and capacity building. However, the use of innovative technology and the gradual digitization of the maritime sector provides a unique advantage. The rise of big data collection and analysis, paired with advancements in machine learning (ML) and AI, as well as the revolution in the earth observation industry, has opened the doors for radical transformation in the maritime sector.
Nonetheless, risks and security threats in the maritime sector are dynamic in nature and technological advancements do not translate to a perfectly safe future. In this ever-changing picture of maritime security, authorities in the maritime sector need real-time actionable intelligence to stay one step ahead of bad actors and safeguard lives, property, and maritime borders. Deriving actionable intelligence from maritime data is a complex task, with millions of data points generated every hour over a vast operational area from an increasing array of sensors, including AIS, earth observation satellites, maritime weather sensors, and other sensor platforms. Extracting intelligence from this proverbial ocean of data requires highly specialized maritime risk subject matter expertise to be combined with the power of cloud computing and AI.
A telling example of the complexity of this task is presented in Park et al. 2020, where AIS data was used along with different forms of satellite imagery, including Synthetic Aperture Radar (SAR), visible infrared, and high-resolution optical imagery, to detect a massive fleet of 900 fishing vessels, operating illegally within North Korean waters. In two years, the fleet captured over 164,000 metric tons of Japanese flying squid worth nearly half a billion dollars, which has resulted in the decline of squid stocks to a quarter of those in 1997. The need for a real-time intelligence gathering platform was also highlighted by the Nord Stream pipeline explosion in 2022, which resulted in over 400,000 tons of methane leaking into the atmosphere and is believed to be a “deliberate and irresponsible act” according to NATO. While retrospective analysis of satellite imagery by other sources shows two dark vessels operating in the area in the days leading up to the incident, the attribution remains uncertain, in-part due to the lack of real-time insights.
The field of AI is at the precipice of bringing transformative change to our society. GSTS provides AI powered solutions that deliver increased productivity and security in the maritime sector through advanced collaboration on a common platform. While the capability of gathering different sources of data is key, the ability to analyse that data in new and innovative ways, and to have strong foundations in machine learning and AI, is what makes OCIANA™ a platform truly capable of delivering tangible value. OCIANA™ translates data into actionable intelligence with the primary objective of making the oceans more safe and secure.
Finding the Signal in a Sea of Data
One of the challenges associated with interpreting maritime data is deciphering mixed and sometimes conflicting signals. Different data sources may be transmitted at a variety of frequencies, and in the case of AIS data, may vary according to details like vessel type, behaviour, and signal density. As well, AIS data may need to be fused with other vessel detection sources to capture vessels of interest, such as RF sensors, optical sensors, and coastal radar systems. The variability inherent in fusing these sensor signals requires a nuanced understanding of their spatial and temporal properties, and their relation to vessel identity and behaviour. In locations with heavy vessel traffic, signal collision can increase the presence of noise in data, which can make it difficult to track particular types of vessels (e.g., small fishing vessels). Such vessels also tend to exhibit more erratic movement patterns, which makes the task of predicting their behaviour more challenging. OCIANA™ has developed novel, advanced capabilities for identifying vessel patterns prior to the occurrence of anomalous events or infractions. Through the explicit detection of spatiotemporal signatures, combined with auxiliary parameters derived from our own maritime intelligence expertise, OCIANA™ can thus surface the critical “patterns of life” associated with maritime threats.
Bridging the gaps of maritime intelligence requires considering the full suite of sensor signals. Terrestrial AIS systems can effectively de-collide messages in highly trafficked coastal waters but fail to detect vessels in open water. Satellite AIS sensor systems support global scale monitoring but may have higher latency due to data transmission. Coastal radar monitoring systems can observe vessels in coastal waters even when AIS transponders are switched off or not present onboard; however, the geographic range of radar systems is limited and impacted by local topography. As well, recorded radar tracks may be affected by weather conditions and the geometric properties of individual vessels. There is no one-size-fits-all solution to maritime threat surveillance – surfacing critical insights requires the fusion and integration of multiple signals using ML/AI.
OCIANA™ has been developed to reduce noise and amplify signal of multiple signal data types. Through the fusion of heterogeneous data sources including ocean sensors, environmental data, satellite and terrestrial AIS, coastal radar, and in some cases, RF signal detectors, GSTS has refined and deployed recent AI advancements to successfully tackle the challenges of maritime threat detection and monitoring.
Coastal Threats Monitoring with OCIANA™
GSTS’ AI-powered platform, OCIANA™ acts as an intelligence layer between the complex web of maritime data and the user, helping to clear the proverbial “fog-of-war” from the maritime picture, and providing concise insights to ensure the safety and security of coastal regions. The platform is capable of ingesting large volumes of data and raising alarms on potential coastal maritime threats in near realtime, i.e., an alarm raised in under 5 minutes when a vessel exhibits behaviour associated with a potential threat.
OCIANA™ works on the principle of establishing normalcy models of vessels and identifying deviations from expected vessel behaviours. The development of normalcy models considers a rich array of ambient conditions including geographical features (e.g., Is the vessel entering or leaving shore at an unknown port of entry?), temporal features (e.g., daily, weekly, and seasonal operating patterns of vessels), and sea conditions. These context-aware normalcy models are then used to identify vessel patterns that do not adhere to any established pattern of normal behaviour, and flagged as anomalous.
A vessel may be considered anomalous under various conditions, such as the speed of the vessel, the spatial properties of the vessel’s movements, and the region in which the vessel is operating. In addition, the normalcy models also account for different combinations of vessel characteristics and ambient conditions to establish normalcy. For example, a vessel operating in shallow waters may be considered normal within its isolated context, a vessel operating at a speed of 8 knots may be considered normal in its isolated context, but a vessel operating in shallow waters at a speed of 8 knots (i.e., both conditions fulfilled simultaneously) may be considered anomalous.
Coastal Threats AI is capable of distinguishing between anomalies that might lead to tangible threats, and benign anomalies that are associated with low or no risk. It incorporates the following principle:
All anomalous vessel behaviours are not maritime threats, but all maritime threats must have an association to anomalous vessel behaviour.
This principle keeps the rate of false alarms raised by the platform to a minimum, ensuring that the maritime intelligence picture is not cluttered with unnecessary information. As well, this capability recognizes that normality is a dynamic state, which must adapt and learn over time to capture trends in maritime activity.
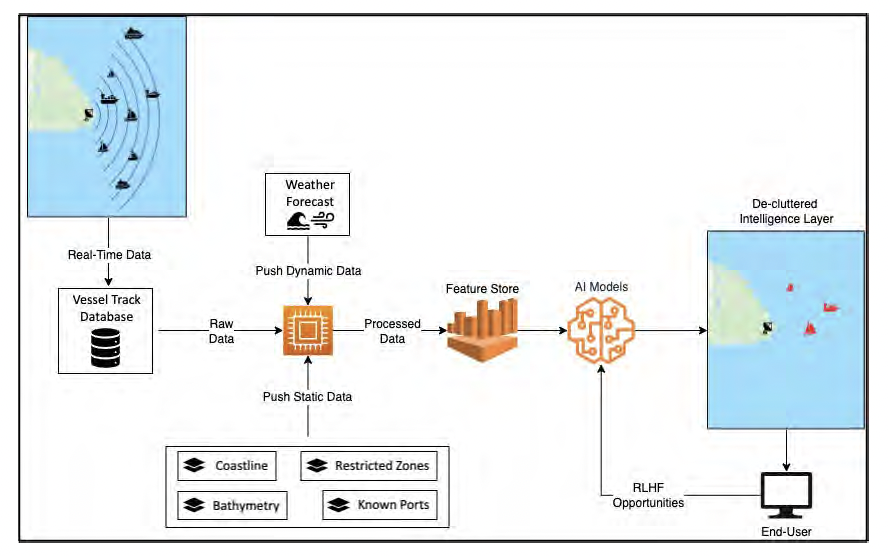
As opposed to a monolithic one-size-fits-all solution, which, in most cases, compromises accuracy, especially in a domain as complex as this, Coastal Threats AI is currently comprised of over 50 deep learning models, each capturing specific context details of vessels, locations, and maritime activity. This web of models remains hidden in the background, and the user is only presented with a single point of interaction with the platform, offering a seamless learning experience for new users.
In addition, extensive care has been taken in packaging OCIANA’s capabilities into a light-weight portable plug-and-play solution, resulting in a straightforward configuration for existing maritime applications. The platform offers significant flexibility to fine-tune the behaviour of the underlying models for unique operational needs. For example, users can tweak certain parameters to make it more or less conservative when identifying deviations from established normalcy patterns, making the platform interoperable between relatively safe waters, and more risk-prone areas. OCIANA™ is also capable of learning and will continuously improve when deployed to a particular region over a period of time, producing lower false alarms, and identifying potential threats that it may have missed earlier. As GSTS develops OCIANA™ further, there are opportunities for Reinforcement Learning from Human Feedback (RLHF) that can have a significant impact on the performance of the platform.
The Coastal Threats AI capability seamlessly integrates with the OCIANA™ platform to leverage its comprehensive maritime data and intelligence extraction features. By leveraging OCIANA’s architecture and AI algorithms, Coastal Threats AI enhances the platform’s ability to process and interpret vast amounts of maritime data. This integration allows for the effective identification of potential coastal threats, ensuring real-time, actionable intelligence for maritime security. Coastal Threats AI is a significant enhancement to OCIANA™, augmenting its capability to provide usercentric, precise, and swift responses to maritime security challenges.
Operationalizing AI for Maritime Safety and Security
The use of ML/AI is already transforming industries around the world, and technology solutions will only get stronger in the future. We believe that the use of these technologies has immense potential in increasing the safety and security of the maritime sector, while also driving the sector towards a more sustainable future. GSTS wields the power of these combined technologies with great responsibility, by creating an internal atmosphere of strong vetting procedures implemented at each development stage, and providing ongoing consideration to the ethical dimensions of working in this space.
One important step taken by GSTS towards the responsible use of AI is to bring a certain level of transparency to the platform through the use of Explainable AI (XAI). The inherent opaqueness of AI platforms can make it difficult to gain the trust of users when it comes to explaining the decisions it takes. We overcome this obstacle by pairing each decision taken by OCIANA™ with a logical explanation of the triggers that were activated for a positive identification of a maritime threat. This approach allows users to gain more confidence in the decisions taken by the platform. It also allows the user to form an opinion on whether they agree or disagree with a decision taken by the platform because they have access to some semblance of the structure. Thus opportunities for continuous improvement of the platform are created through human-in-the-loop reinforcement learning.
As the maritime sector moves into a more technologically advanced future, it must be emphasized that bad actors will have access to a similar set of platforms that can be used to outsmart and exploit existing safeguards. Early adoption of AI platforms in the day-to-day operations of the maritime sector is a win-win scenario because not only does it protect assets today, but it also makes these technologies better when they learn from long-term patterns in the data. A maritime user that adopts an AI platform early will benefit from the fact that the models deployed within their ecosystem will eventually be trained to respond to their unique needs having learned from their unique data and operational uses.
To learn more contact us today: sales@gsts.ca